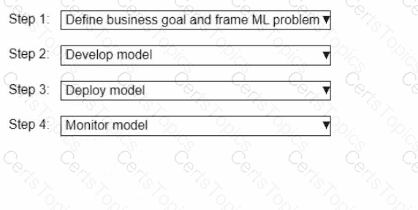
Building a well-architected ML workload follows a structured lifecycle as outlined in AWS best practices. The process begins with defining the business goal and framing the ML problem to ensure the project aligns with organizational objectives. Next, the model is developed, which includes data preparation, training, and evaluation. Once the model is ready, it is deployed tomake predictions in a production environment. Finally, the model is monitored to ensure it performs as expected and to address any issues like drift or degradation over time. This order ensures a systematic approach to ML development.
Exact Extract from AWS AI Documents:
From the AWS AI Practitioner Learning Path:
"The machine learning lifecycle typically follows these stages: 1) Define the business goal and frame the ML problem, 2) Develop the model (including data preparation, training, and evaluation), 3) Deploy the model to production, and 4) Monitor the model for performance and drift to ensure it continues to meet business needs."
(Source: AWS AI Practitioner Learning Path, Module on Machine Learning Lifecycle)
Detailed Explanation:
Step 1: Define business goal and frame ML problemThis is the first step in any ML project. It involves understanding the business objective (e.g., reducing churn) and framing the ML problem (e.g., classification or regression). Without this step, the project lacks direction. The hotspot lists this option as "Define business goal and frame ML problem," which matches this stage.
Step 2: Develop modelAfter defining the problem, the next step is to develop the model. This includes collecting and preparing data, selecting an algorithm, training the model, and evaluating its performance. The hotspot lists "Develop model" as an option, aligning with this stage.
Step 3: Deploy modelOnce the model is developed and meets performance requirements, it is deployed to a production environment to make predictions or automate decisions. The hotspot includes "Deploy model" as an option, which fits this stage.
Step 4: Monitor modelAfter deployment, the model must be monitored to ensure it performs well over time, addressing issues like data drift or performance degradation. The hotspot lists "Monitor model" as an option, completing the lifecycle.
Hotspot Selection Analysis:
The hotspot provides four steps, each with the same dropdown options: "Select...," "Deploy model," "Develop model," "Monitor model," and "Define business goal and frame ML problem." The correct selections are:
Step 1: Define business goal and frame ML problem
Step 2: Develop model
Step 3: Deploy model
Step 4: Monitor model
Each option is used exactly once, as required, and follows the logical order of the ML lifecycle.
[References:, AWS AI Practitioner Learning Path: Module on Machine Learning Lifecycle, Amazon SageMaker Developer Guide: Machine Learning Workflow (https://docs.aws.amazon.com/sagemaker/latest/dg/how-it-works-mlconcepts.html), AWS Well-Architected Framework: Machine Learning Lens (https://docs.aws.amazon.com/wellarchitected/latest/machine-learning-lens/), , , ]