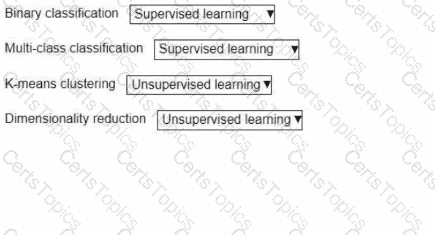
The company is developing ML applications for various use cases, and the task is to select the correct ML paradigm (supervised or unsupervised learning) for each. Supervised learning involves training a model on labeled data to make predictions, while unsupervised learning identifies patterns or structures in unlabeled data. Each use case aligns with one of these paradigms based on its requirements.
Exact Extract from AWS AI Documents:
From the AWS AI Practitioner Learning Path:
"Supervised learning uses labeled data to train models for tasks like classification (e.g., binary or multi-class classification), where the model predicts a category. Unsupervised learning works with unlabeled data for tasks like clustering (e.g., K-means clustering) or dimensionality reduction, identifying patternsor reducing data complexity without predefined labels."
(Source: AWS AI Practitioner Learning Path, Module on Machine Learning Strategies)
Detailed Explanation:
Binary classification: Supervised learningBinary classification involves predicting one of two classes (e.g., yes/no, spam/not spam) using labeled data, making it a supervised learning task. The model learns from examples where the correct class is provided.
Multi-class classification: Supervised learningMulti-class classification extends binary classification to predict one of multiple classes (e.g., categorizing items into several groups). Like binary classification, it requires labeled data, so it falls under supervised learning.
K-means clustering: Unsupervised learningK-means clustering groups data into clusters based on similarity, without requiring labeled data. This is a classic unsupervised learning task, as the algorithm identifies patterns in the data on its own.
Dimensionality reduction: Unsupervised learningDimensionality reduction (e.g., using techniques like PCA) reduces the number of features in a dataset while preserving important information. It does not require labeled data, making it an unsupervised learning task.
Hotspot Selection Analysis:
The hotspot lists four use cases, each with a dropdown containing "Select...," "Supervised learning," and "Unsupervised learning." The correct selections are:
Binary classification: Supervised learning
Multi-class classification: Supervised learning
K-means clustering: Unsupervised learning
Dimensionality reduction: Unsupervised learning
Each paradigm (supervised and unsupervised learning) is used twice, as the question allows for paradigms to be selected one or more times.
[References:, AWS AI Practitioner Learning Path: Module on Machine Learning Strategies, Amazon SageMaker Developer Guide: Supervised and Unsupervised Learning (https://docs.aws.amazon.com/sagemaker/latest/dg/algos.html), AWS Documentation: Introduction to Machine Learning Paradigms (https://aws.amazon.com/machine-learning/), , ]