AWS Certified Data Analytics - Specialty DAS-C01 Dumps Questions and Answers
Exam Code:
DAS-C01
Exam Name:
AWS Certified Data Analytics - Specialty
Certification:
AWS Certified Data Analytics
Vendor:
Amazon Web Services
Exam Questions:
207
Last Updated:
Jul 27, 2024
Exam Status:
Stable
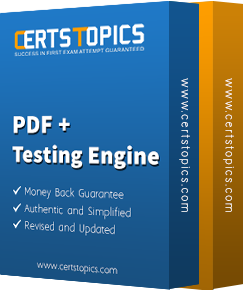
Amazon Web Services AWS Certified Data Analytics DAS-C01 Exam Dumps
CertsTopics offers Amazon Web Services AWS Certified Data Analytics - Specialty real exam questions and practice test engine with real questions and verified answers. Try AWS Certified Data Analytics - Specialty exam questions for free. You can also download a free PDF demo of Amazon Web Services AWS Certified Data Analytics - Specialty exam. Our AWS Certified Data Analytics - Specialty Combo Package for which includes PDF (Printable Format) and Testing Engine (Works on Windows and MAC) which ensures you to go through all certification topics and provides you ultimate satisfaction to pass your exam in your first attempt.